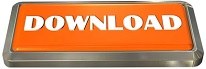
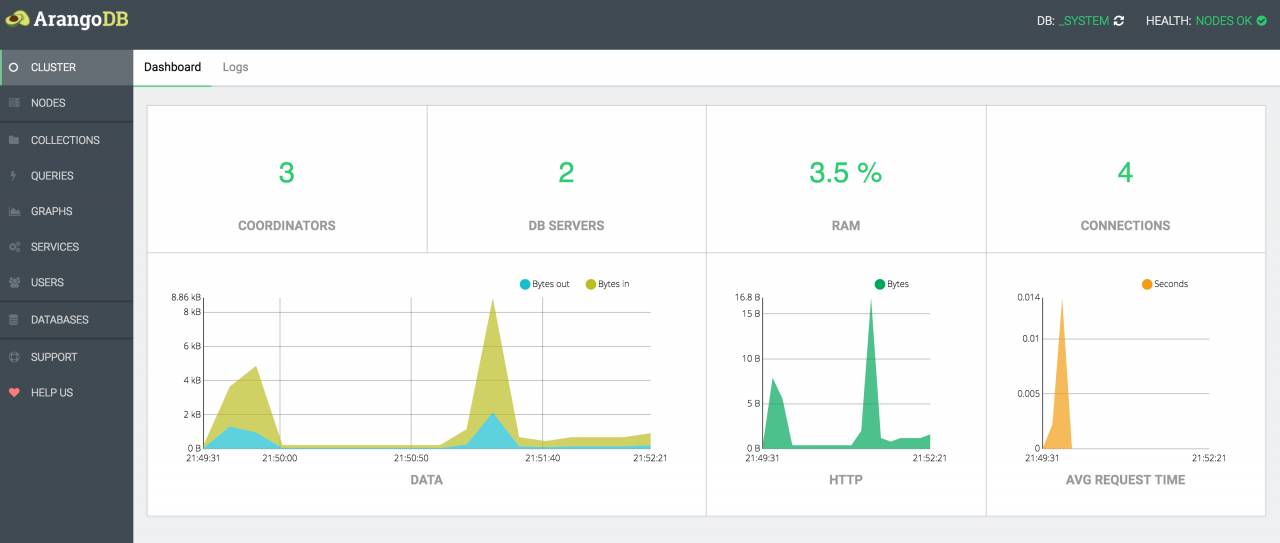
In recent years, graphs have been shown increasingly important to big data applications such as social network analysis, spatiotemporal analysis and navigation, and consumer analytics, as it is able to capture complex relationships and data dependencies. Even until now, RDBMSs still remain as mainstreaming data management systems. The relational data model achieves great success to support a wide spectrum of applications that are related to financial, personnel, manufacturing, and logistical data management. Since then, various RDBMSs have been developed with standard SQL to support data definition, data manipulation, and data control operations. Codd introduced the relational data model with relations to represent data, with relational algebra and relational calculus to operate data, and relational integrity constraint to control the consistency and completeness of data. We then conduct extensive experiments to evaluate them and make the following findings: (1) RDBMSs outperform GDMBSs by a substantial margin under the workloads which mainly consist of group by, sort, and aggregation operations, and their combinations (2) GDMBSs show their superiority under the workloads that mainly consist of multi-table join, pattern match, path identification, and their combinations.Į.F. For this reason, in this paper, we first extend a unified benchmark for RDBMSs and GDBMSs over the same datasets using the same query workload under the same metrics. As pointed out in the existing literature, both RDBMSs and GDBMSs are capable of managing graph data and relational data however, the boundaries of them still remain unclear.
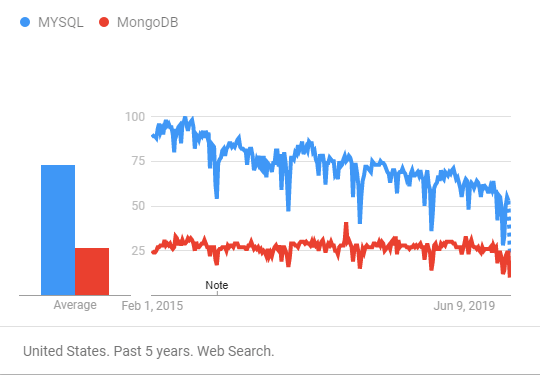
Recently, due to the variety properties of big data, graph database management systems (GDBMSs) have emerged as an important complement to RDBMSs. Over decades, relational database management systems (RDBMSs) have been the first choice to manage data.
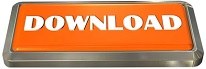